Disease Forecasting
Disease forecasting in agriculture refers to the act of predicting the occurrence of plant diseases in a specific area in advance, allowing for proactive measures to prevent losses. These forecasts are essentially anticipations of potential disease outbreaks or the escalation of disease severity. This process requires effective teamwork and significant investment of time, resources, and funds. Disease forecasting serves as a valuable tool for ensuring timely application of chemicals and other control measures.
One of the earliest examples of such forecasting services was established in the 1920s in France, Germany, and Italy, where grapevine downy mildew forecasting schemes were implemented. Various disease forecasting methods are currently available for a range of plant diseases.
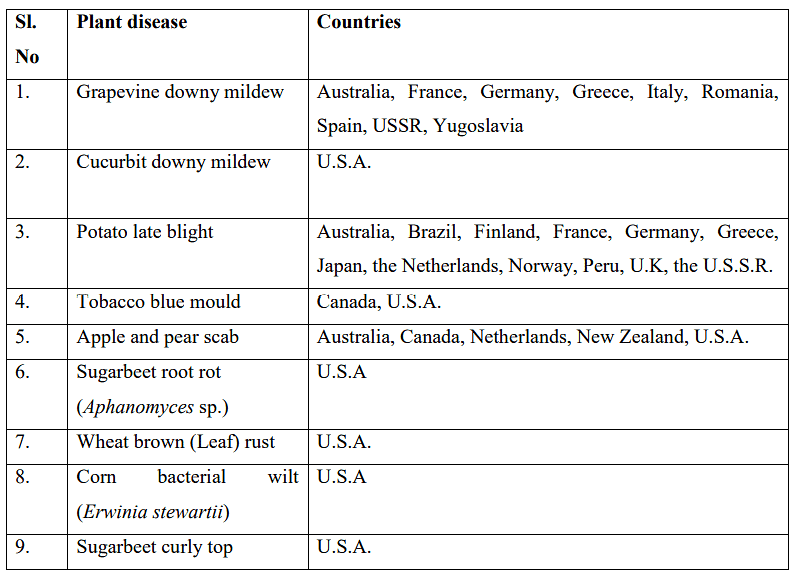
Forecasting diseases is a crucial aspect of applied epidemiology, which involves understanding how diseases develop based on various factors related to the host and pathogen. To accurately predict disease outbreaks, it's essential to have knowledge of the elements that contribute to an epidemic in advance.
Here are the types of information required for disease forecasting:
Host Factors
- Prevalence of susceptible plant varieties in the specific area.
- How the host responds to the pathogen at different growth stages. Some diseases affect seedlings, while others target mature plants.
- The density and distribution of the host population in the area. Dense populations of susceptible varieties can lead to rapid disease spread. Planting susceptible varieties in scattered locations within a limited area is less likely to result in an epidemic.
Pathogen Factors
- The quantity of primary (initial) inoculum present in the air, soil, or planting material.
- How the inoculum is dispersed.
- The process of spore germination.
- How infection occurs.
- The incubation period of the disease.
- Spore production on infected host plants.
- The re-dispersal or dissemination of spores.
- The presence of overwintering or perennating stages of the pathogen.
- The potential for and density of inoculum in seeds, soil, and the air.
Environmental Factors
- Temperature conditions in the area.
- Humidity levels in the environment.
- Intensity of light.
- Wind speed in the region.
Having comprehensive information about these factors is essential for making accurate disease forecasts, as they play critical roles in the development and spread of plant diseases.
Requirements or conditions for disease forecasting
There are five primary conditions that must be met to ensure the effectiveness and success of disease forecasting:
- The disease in question must cause significant economic harm in terms of reduced yields or compromised quality. Evaluating the extent of damage is crucial for developing strategies to control the disease. For example, annual assessments have shown that barley powdery mildew (Erysiphe polygoni) in England and Wales can lead to yield losses ranging from 6% to 13%. If potato late blight progresses to 75% severity by mid-August, it can result in a 28% yield loss. Diseases like apple scab and potato common scab not only reduce crop quality but also lead to substantial financial losses for growers.
- Control measures for the disease must be available at a cost that is economically feasible for growers.
- The disease should exhibit variability in the timing of its initial infections and the rate at which it progresses from one season to another. If the disease behaves consistently without seasonal variation, there may be no need for forecasting.
- The criteria or models used for making predictions must be based on well-founded research conducted in both laboratory and field settings. These models should be rigorously tested over several years to ensure their accuracy and applicability across various locations where they are intended to be used.
- Growers must have access to an adequate workforce and the necessary equipment to implement control measures when disease warnings are issued. Long-term forecasts or predictions are generally more valuable than short-term ones.
In summary, for disease forecasting to be effective, the disease must be economically significant, control measures must be cost-effective, disease behavior should vary seasonally, prediction models must be well-researched and tested, and growers must have the resources to take action based on the forecasts.
|
Download the notes
Modelling and Disease forecasting; Plant quarantine
|
Download as PDF
|
Methods of Disease Forecasting
Disease forecasting involves a combination of field observations, weather data collection, examination of crop varieties, and investigations with correlations.
Typically, the following methods are used for disease forecasting:
- Forecasting Based on Primary Inoculums: This approach involves assessing the presence, density, and viability of primary inoculum (the initial source of disease-causing agents) in the air, soil, or planting material. Various methods, including spore traps, are used to detect viable spores or propagules in the air. For soil-borne diseases, monoculture techniques are employed to determine the primary inoculum in the soil. Random seed testing methods can also identify diseases like loose smut in wheat, ergot in pearl millet, and viral diseases in potato seeds. Additionally, some virus diseases are influenced by the severity of the preceding winter, which affects the vector population in the growing season, such as Sugarbeet yellows virus.
- Forecasting Based on Weather Conditions: Weather conditions, including temperature, relative humidity, rainfall, light, wind velocity, and more, are monitored during the crop season and intercrop periods. These conditions are recorded both above the crop and at the soil surface.
- Forecasting Based on Correlative Information: Historical weather data from multiple years are collected and correlated with disease intensity. By comparing this data, disease forecasts are generated. For example, forecasting criteria are developed based on the comparison of disease observations with standard meteorological data for diseases like Septoria leaf blotch of wheat, fire blight of apple, and barley powdery mildew.
- Computer-Based Disease Forecasting: In advanced countries, computers are used for disease forecasting. An example is the "Blitecast" program in the USA, which predicts potato late blight. It utilizes data on relative humidity and minimum temperature to provide rapid and accurate results.
- Specific Disease Forecasting Programs: Various countries have developed disease-specific forecasting programs, including:
- Forecasting early and late leaf spots of groundnut in the USA, based on specific conditions like leaf wetness duration and minimum temperature.
- Forecasting late blight of potato in the USA, where the initial appearance of late blight is predicted after a certain number of blight-favorable days.
- Predicting epidemics of blister blight of tea in India, considering spore count in the air and leaf surface wetness duration.
- Forecasting Southern corn leaf blight in the USA using a conceptual model.
- Predicting rice blast in India based on minimum night temperature and high relative humidity, with computer-based forecasting systems also available.
- Wheat Stem Rust Forecasting: Wheat stem rust epidemics are predicted by analyzing rain samples to determine the presence of inoculum in the air. Wind trajectories are prepared to monitor air-borne primary inoculum and its deposition.
- Brown Stripe Downy Mildew of Corn Forecasting: This disease, specific to India, is forecasted based on average rainfall of 100 to 200 cm or more, accompanied by low temperatures (25°C or less).
- Spore Trapping: Spore trapping is crucial for gathering biological data to develop consecutive forecasting models. Different spore traps, such as Hirst's volumetric spore trap, Rotorod samplers, Anderson cascade spore samplers, and others, are used to sample fungal spores. This method helps understand disease epidemiology, pathogen behavior, and aids in developing disease management strategies.
In summary, disease forecasting relies on various methods, including primary inoculum assessment, weather data analysis, historical correlations, computer models, and disease-specific programs, to help agricultural communities anticipate and mitigate disease outbreaks effectively.
|
Take a Practice Test
Test yourself on topics from UPSC exam
|
Practice Now
|
Cylindrical rods or microscopic glass slides
Various methods and devices are employed for collecting fungal spores in disease forecasting.
Here are descriptions of some of these methods:
- Cylindrical Rods or Microscopic Glass Slides: These are used to gather data on spore presence in a specific location. In this method, a microscopic slide is coated with a sticky grease to capture spores. However, it's important to note that this method doesn't allow for accurate quantitative estimation due to the low number of spores collected.
- Hirst's Volumetric Spore Trap (Hirst 1952): This instrument functions by drawing air at a controlled rate and directing it onto a glass slide that moves using a clockwork mechanism past an opening. It continuously counts spores over a 24-hour period, enabling the calculation of spore concentration per unit volume of air at any given time.
- Rotorod Sampler or Rotorod Spore Trap (Sutton and Jones 1976): This device consists of a 'U'-shaped rod attached to the midpoint of a small battery-operated electric motor. The rod's surface is covered with a strip of transparent cellophane coated with Vaseline to capture spores. By measuring the strip's area and the rotation speed, the volume of air sampled can be determined.
- Anderson Cascade Spore Sampler: This device uses Petri plates containing nutrient agar to collect spores from the air.
- Bourdillon Slit Sampler: Air is drawn into a chamber by a vacuum pump, and it strikes a rotating Petri dish containing agar medium. The agar medium captures the spores present in the air. Afterward, the concentration of viable spores is calculated by counting germinated spores in the medium.
- Burkard's 7-Day Volumetric Spore Trap: This device records spore counts in the air using a pump over a 7-day period. Spores are collected on a cellophane strip wrapped around a drum rotating inside a chamber.
- Jet Spore Trap: In the previously mentioned sampling methods, it is not possible to determine the viability of the spores collected. To address this limitation, living plants are sometimes used as spore traps. A jet spore trap works by impacting spores in an air jet into a column of still air, causing them to fall and settle on leaf segments exposed at the base of the chamber. This method allows for the determination of the number of viable spores.
In summary, these various spore trapping methods are employed in disease forecasting to collect fungal spores from the air and assess their concentration and viability, which is crucial for understanding disease epidemiology and behavior and for developing disease management strategies.